Intelligent Tutoring Systems: Data is the New Oil #AIEd
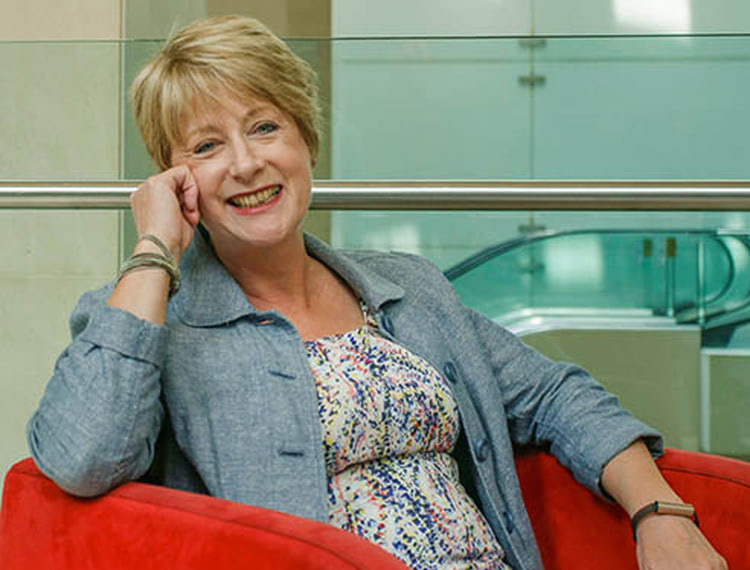
AI and Education: the Reality and the Potential
While I was enjoying my morning latte on the tube earlier this month, I spotted this headline in the New Scientist: AI achieves its best ever mark on a set of English exam questions: i.e. the knowledge-based curriculum and exams.
This is significant in three important ways, and these are also the three ways that I want to discuss AI and education with you.
- Firstly, it demonstrates the power of the AI that we can build to learn and to teach what we currently value in our education systems. This speaks to my first point that will be about the way AI can support teaching and learning.
- Secondly, if this is headline news, then it demonstrates that we do not know enough about AI, because passing an exam is a very AI type problem to solve, and we should not be surprised that AI can do this. It should be something we take for granted, because we all understand enough about AI to know the basics of what it can and cannot achieve.
- Thirdly, this headline draws our attention to the fact that we can build AI that can achieve what we set our students to achieve. The AI will get better and faster at this and it therefore is not intelligent to continue to educate humans to do what we can automate. We need to change our education systems to value our rich human intelligence. This need to change what and how we teach is also connected with the way that AI powers the automation that is changing our lives at some pace. We need very different skills, abilities and intelligences to thrive in the modern world.
One only has to look at our current political failure in the UK, to see that the much-heralded education that we have provided for the last century has not provided our politicians with the emotional and social intelligence and the ability to solve problems collaboratively that the modern world requires.
AI is empowering automation and the Fourth Industrial Revolution and its impact on education will be transformative.
What is this thing called AI?
A basic definition of AI is one that describes it as ‘technology that is capable of actions and behaviours that require intelligence when done by humans’.
We may think of it as being the stuff of science-fiction, but actually it’s here and with us now from the voice-activated digital assistants that we use on our phones and in our homes, to the automatic passport gates that speed our transit through airports and the navigation apps that help us find our way around new cities and cities that we know quite well.
We use AI every day, probably without giving it a thought.
The desire to create machines in our own image is not new, we have, for example, been keen on creating mechanical ‘human’ automata for centuries.
However, the concept of AI was really born 63 years ago in September 1956 when 10 scientists at Dartmouth College in New Hampshire spent the summer working to create AI.
If we look at the premise for this two-month study, we see that it is a premise that believes that: ‘every aspect of learning or any other feature of intelligence can in principle be so precisely described that a machine can be made to simulate it.’
And, although it seems incredibly arrogant now, the belief was that over a two-month period the team of scientists, would be able to make ‘a significant advance … in one or more of these problems.’
Some early successes
Following on from this there were some early successes. For example, expert systems that were used for tasks such as diagnosis in medicine.
These systems were built from a series of rules through which the symptoms a patient presented with could be matched to potential diseases or causes, so enabling the doctor to make a decision about treatment.
These systems were relatively successful, but they were limited because they could not learn.
All of the knowledge that these expert systems could use to make decisions had to be written at the time the computer program was created.
If new information was discovered about a particular disease or its symptoms, then if this was to be encompassed by the expert system it’s rule-based had to be changed.
In the 1980s and 90s we had built useful systems, but certainly we were not anywhere near the dreams of the 1963 Dartmouth College conference.
We plunged into what has been described as an AI winter where little significant progress was made and disappointment was felt by those who had such high expectations of what could be achieved.
Game changing breakthrough
Then in March 2016 came a game changing breakthrough. A breakthrough that was based on many years of research.
A breakthrough that was made when Google Deepmind produced the AI system called AlphaGo that beat Lee Sedol, the world ‘Go’ champion. This was an amazing feat.
A feat that could seem like magic, and whilst many of the techniques behind these machine learning algorithms are very sophisticated, these systems are not magic and they have their limitations.
Smart as AlphaGo is, the real breakthrough was due to a combination that one might describe as a perfect storm.
This perfect storm arises due to the combination of:
- our ability to capture huge amounts of data,
- combined with the development of very sophisticated AI machine learning algorithms,
- plus affordable competing power and memory.
These three factors when combined provide us with the ability to produce a system that could beat the world Go champion.
Data is the new oil
Each of the elements in that perfect storm: the data, the sophisticated AI algorithms and the computing power and memory are important, but it is the data that has captured the imagination. And that has led to claims that ‘data is the new oil’, because it is the power behind AI and AI is a very profitable business, just like oil.
However, it’s important to remember, that just like oil, data is crude and it must be refined in order to derive its value.
It must be refined by these AI algorithms. But even before the data can be processed by these algorithms it must be cleaned.
So just like oil, there is a lot of work that needs to be done on the data before its value can be reaped. And even when we do reap this value from the data, it’s important to remember that machine learning is still basically just a form of pattern matching.
Machine learning is certainly smart, very smart indeed, but it cannot learn everything.
Limitations of AI
AI has its limitations. For example, AI does not understand itself and struggles to explain the decisions that it makes. It has no common sense.
If I ask you the audience this evening these questions:
- Are you an empathetic friend?
- How well do you understand quantum physics?
- How are you feeling right now?
- Can you meditate?
You will not struggle to answer, but AI would. So, the first important point to remember is that humans are intelligent in many ways.
AI and Human Intelligence (HI) are not the same and the differences are extremely important, it is true that we have built our AI systems to be intelligent in the way that we perceive value in our human intelligence.
Grandmaster level Chess-playing AI
I remember in the early days of studying AI, the first grandmaster level Chess-playing Computer had been built and had beaten world champion Garry Kasparov.
This seemed an amazing feat and there were people who thought that having cracked chess, which could be described as the pinnacle of human intelligence, intelligent people play chess after all.
It was thought that we had cracked intelligence. And then people realised that the abilities that we take for granted, such as the ability to see, are far harder to achieve than is playing chess.
Decades later, we have managed to build AI systems that can see, to an extent, but they still have their limitations.
What we need if we are to progress and grow our human intelligence, is to make sure that we recognise the need for humans to complement AI, not to mimic and repeat what the AI can do faster and more consistently than we can.
What are the implications for education?
And so, what are the implications: the potential and the reality of AI within education?
I believe that it is useful to think about this question from three perspectives:
- Using AI in education to tackle some of the big educational challenges;
- Educating people about AI so that they can use it safely and effectively;
- Changing education so that we focus on human intelligence and prepare people for an AI augmented world.
It is important to recognise that these three elements are not mutually exclusive. In fact, they are far from being mutually exclusive. They are interrelated in important ways.
Using AI in education to tackle some of the big educational challenges.
Challenges such as the achievement gaps we see between those who achieve well educationally and those who do not. And challenges, such as those posed by learners with special and particular learning needs.
If we start by looking at the reality of the systems that are available here and now, to help us tackle some of these challenges, then we will see the beginnings of the potential for the future.
Data, AI and computing power in education – intelligent platforms and intelligent tutoring systems
Data has been a game changer when it comes to educational AI. And that’s true for US companies, and here in the UK too.
If we take the London-based Century Tech, they have developed a machine learning platform that can personalise learning to the needs of individual students across curriculum areas to help them achieve their best.
Their machine learning is informed by what we understand from neuroscience about the way the human brain learns.
A further reality is that, in addition to being able to build intelligent platforms, such a Century, we can build intelligent tutors that can provide individual instruction to students in a specific subject area.
These systems are extremely successful, not as successful as a human teacher who is teaching another human on a one-to-one basis, but the AI can, when well-designed, be as effective as a teacher teaching a whole class of students.
Building cognitive fitness
In addition to intelligent platforms and intelligent tutoring systems, there are many intelligent recommendation systems that can help teachers to identify the best resources for their students to use, and that help learners identify exactly what materials are most suitable for them at any particular moment in time.
It is not just by learning particular areas of the curriculum that AI can make a big difference.
AI can also help us to build our cognitive fitness, so that we have good executive functioning capabilities, so that we can pay attention when needed, remember what we learn and focus on what needs to be done.
This system called MyCognition, for example, enables each person who uses it to complete a personal assessment of their cognitive fitness and then train themselves using a game called Aquasnap.
AI helps Aquasnap to individualise training according to the needs of the particular person who is playing.
Finally, just in case you thought the reality of AI was only for adults, think again.
This example from Oyalabs is a room-based monitor that can track the progress of a baby and provide that baby’s parents with individual supportive feedback to help them support their child’s development as effectively as possible.
Rose Luckin, Professor of Learner Centred Design, UCL Knowledge Lab
First published on The Knowledge Illusion, this article reviews AI and Education: the Reality and the Potential
In this first article “Intelligent Tutoring Systems: Data is the New Oil” Rose covers the way AI can support teaching and learning.
The next article, “Educating people about Artificial intelligence, so that they can use it safely and effectively” dicusses helping people understand enough about AI to know the basics of what it can and cannot achieve.
Finally Rose will explore how we need to change our education systems to value our rich human intelligence.
Responses