Preparing for an #AI world: Changing education to focus on human intelligence
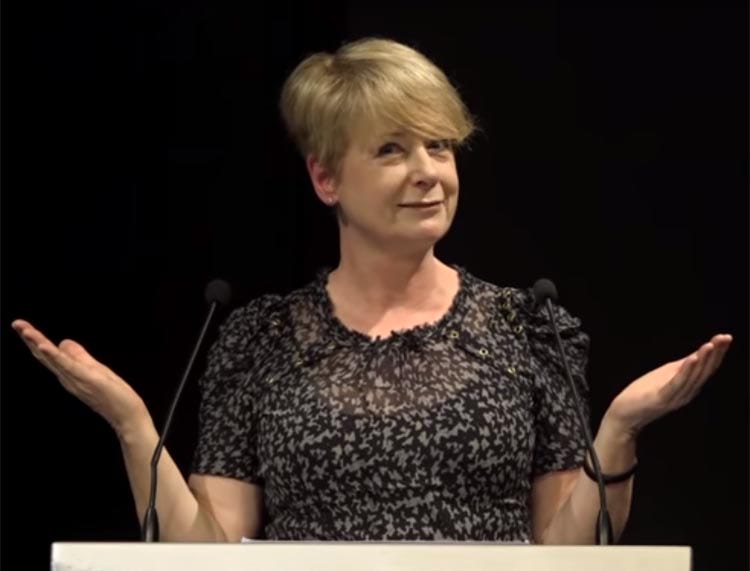
Many people, including the World Education Forum are telling us that we are now entering the Fourth Industrial Revolution – the time when many factors across the globe, including the way that AI is powering workplace automation, are changing the workplace and our lives for ever.
There is much media attention to this Fourth Industrial Revolution with some coverage making such positive predictions as these from Australia that suggest that we will have two hours more time each week, because some of the more tedious aspects of our jobs will be automated.
Our workplaces will be safer, and jobs will be more satisfying as we learn more.
Not everyone is as optimistic and there are an increasing number of reports that consider the consequences for jobs of the increased automation taking place in the workplace.
We can see from the report called ‘Will robots really steal our jobs’ (PWC 2018), that transportation and storage appear to be the areas of the economy where most job losses will occur.
Education will be the least prone to automation
We could interpret that as meaning that education will not change. However, I believe that education will change dramatically.
It will change as we use more AI and it will change as what we need to teach changes in order to ensure that our students can prosper in an AI augmented world.
The impact will not be felt by everyone equally, those with higher education levels will be least vulnerable when it comes to automation and job loss. We therefore need to provide particular support for those with lower levels of education.
Personally, I do not think all these reports are that useful, interesting as they are. As a race, we humans are rather poor at prediction and the differences of opinion across the different reports indicates the complexity of predicting anything in such fast-changing circumstances.
Trying to work out what to do for the best in a changing world, is a little bit like driving a car in dense fog along a road that you don’t know.
In these circumstances, a map about the road ahead has limited use. What we really need is to know that we have a car that is well-equipped, we have brakes that work, lights that work.
We want to be warm and we want to know that as a driver, we understand how to operate the car, that we understand the rules of the road, that we have eyesight that’s good enough to help us to see in the limited visibility ahead and that we can hear what is going on so that you can spot any impending dangers if they are indicating their presence by being noisy. A huge truck thundering towards us, for example.
So, what’s the equivalent of this good car and good driver when it comes to what we need in order to find our way through the fog of uncertainty around the Fourth Industrial Revolution?
This is a subject that I have studied and written about quite a lot and a subject that is covered in this book: Machine Learning and Human intelligence: the future of education 21stcentury.
The 7 types of intelligence we need humans to develop
Here I can only skim over the way that I unpack the intelligence that we need humans to develop if we are to find our way through this foggy landscape.
This is the intelligence that can help us to cope with the uncertainty and it can help us to differentiate ourselves from AI systems.
This is an interwoven model of intelligence that has seven interacting elements:
1. Interdisciplinary Academic Intelligence
The first element of this interwoven intelligence is: interdisciplinary academic intelligence. This is the stuff that is part of many education systems at the moment.
However, rather than considering it through individual subject areas as we do now, we need to consider it in an interdisciplinary manner. Complex problems are rarely solved through single disciplinary expertise, they require multiple experts to work together.
The world is now full of complex problems and we need to educate people to be able to tackle these complex problems effectively. We therefore need to help our students see the relationships between different disciplines.
We need them to be able to work with individuals who have different subject expertise and to synthesise across these disciplines to solve complex problems.
2. Meta knowing: Understanding what knowledge is
Secondly, we need to help our students understand what knowledge is, where it comes from, how we identify evidence that is sound enough to justify that we should believe that something is true. I refer to this as meta knowing, but of course we can use the terminology of epistemology and personal epistemology to describe this meta knowing.
3. Social intelligence
The third elements of our intelligence that we really need to develop in very sophisticated ways is social intelligence. It is very hard for any artificially intelligent systems to achieve social intelligence, and it is fundamental to our success. Because, we need to collaborate increasingly in order to solve the kinds of complex problems that we will be faced with on a daily basis.
4. Meta cognitive intelligence
Fourthly, we need to develop our meta cognitive intelligence. This is the intelligence that helps us to understand what we need to know to understand how we learn, how we can control our mental processes, how we can maintain our focus and spot when our attention is skidding away from what it is we are trying to learn. These metacognitive processes are fundamental to sophisticated intelligence and they are again hard for AI to achieve.
5. Meta emotional intelligence
The fifth element of intelligence we must consider is our meta emotional intelligence. This is what makes us human. We need to understand the subjective emotional experiences we sense and we need to understand the emotional perspectives of the others with whom we interact in the world. This emotional intelligence is also hard for AI. AI can simulate some of this, but it cannot actually feel and experience these emotions.
6. Meta contextual intelligence
We also need to recognise the importance of our physical presence in the world and the different environments with which we interact. We as humans, are very good at working out how to interact intelligently in multiple different environments. This meta contextual intelligence is something at which we can excel, and something that AI has great trouble with. Context here means more than simply physical location it means location, it means the people with whom we interact, the resources that are available to us and the subject areas that we need to acquire and apply in order to achieve our goal.
7. Accurate perceived self-efficacy
If we can build these interwoven elements of our human intelligence then we can really achieve what’s important for the future of learning and that is: accurate perceived self-efficacy. By this I mean that we can see how we can be effective at achieving a particular goal, at identifying what that goal consists of, identifying what aspects of that goal we believe we can achieve now, what aspects we need to learn about and train ourselves to achieve. In order to be self-effective, we must understand than to apply all the elements of intelligence so that we can work across and between multiple disciplines with other people with effective control and understanding of our mental and emotional processes.
Measuring Human Intelligence
Let me take a moment to stress something important here. This is about intelligence. It is not about 21stcentury skills or so-called softskills.
It is about something much more foundational than any skill or knowledge. It is about our human intelligence.
I also want to emphasize that we can measure the development of our intelligence across all seven elements.
They can all be measured and importantly they can all be measured in increasingly nuanced ways through the use of AI. This enhanced and continual formative assessment of our developing intelligence will shed light on aspects of intelligence and humanity that we have not been able to evidence before.
We can use our AI, to help us to be more intelligent, and this is very important.
The truth of the matter is that being human is extremely important. The very aspects of our humanity, the aspects that we do not measure, but that are fundamental to what it means to be human, are the ones that we are likely to need more of in the future. For example, empathy, love and compassion.
In the words of Yuval Noah Harare from 21 Lessons for the 21stCentury: …” if you want to know the truth about the universe, about the meaning of life, and about your own identity, the best place to start is by observing suffering and exploring what it is.”
What AI can or will ever be able to do this? It is important that we ensure that we still can.
How do those feelings impact upon the way you might behave?
We undervalue these aspects of humanity when it comes to our evaluations of intelligence, and yet I would suggest that it is our human emotional and meta intelligence that enables us to feel horror at human suffering and pleasure at human love.
The holistic set of interwoven intelligence enables us to be human and AI can help us to both develop the sophistication of our intelligence, across all its elements, and it will also help us to assess, and yes, to measure much of this.
If that is what we want to do. What we need is good data, and smart AI algorithms that have been designed in a way that is informed by what we know about how humans learn.
We can collect data from a vast array of sources these days. We can collect it as people interact in the world, even when they do not realise, they are interacting with technology.
We are no-longer simply restricted to collecting data explicitly through the interfaces of our desktop, laptop, tablet and smart phone technologies. We can collect it through observation, wearable technology and facial recognition.
For example, in research conducted at the Knowledge Lab with my colleague Dr Mutlu Cukurova, we collected a range of data in our attempts to understand how we could identify signifiers of collaborative problem-solving efficacy that would be susceptible to AI collection and analysis. We were able to capture, it is complex and does not tell us anything of great interest.
However, by using learning from the social sciences that provides evidence that factors, such as the synchrony of individual group members’ behaviours can signify positive collaborative behaviours.
We can then inform the design of the AI algorithms that we use to process this data. We therefore analysed the eye tracking and hand movement data that we collected through this research test rig.
We found that there was indeed a greater instance of synchrony of eye gaze and hand movements between different members of a group, when that group was behaving in a positively collaborative manner, as assessed by an independent expert.
This is just one example of a small signifier, but when combined with a battery of other detailed signifiers, we can start to generate accurate and nuanced accounts of what is happening as people learn.
Accounts that can be extremely useful to teachers and learners. AI can help us to track and support the development of our human intelligence in very sophisticated ways.
But, what does this mean for teaching?
Numeracy and literacy, including data literacy, will of course remain fundamental to all education, as will the basics of AI;
Emphasis for the remaining subject areas needs to be on what these subjects are, how they have arisen, why they exist, how to learn them and how to apply them to solve complex interdisciplinary problems;
Debate and Collaborative Problem Solving provide powerful ways to help students understand their relationships to knowledge and to hone their ability to challenge and question;
To ensure that teachers and trainers have the time to work with their students and trainees to develop these complex skills, we can use AI to:
- Provide tutoring for numeracy, literacy (including data literacy) and basic subject knowledge;
- We then blend this with our human-intelligent teachers who can refine this understanding through activities such as debate and collaborative problem solving; and to develop learners’ social and meta intelligence (meta-cognitive, meta subjective, meta contextual and accurate perceived self-efficacy);
- And then the finesse to this piece – we use the AI to analyse learner and learning data, so that teachers know when to provide optimal support and learners get to know themselves more effectively.
I find that decision makers in education are very risk averse and often do not want to make big changes, because they are concerned that such changes might disadvantage the people in the process of their education when the change hits.
I can understand this. However, if we do not make big changes the consequences are likely to be worse, and the risks much greater.
As the FT expressed this in 2017:
“The risk is that the education system will be churning out humans who are no more than second-rate computers, so if the focus of education continues to be on transferring explicit knowledge across the generations, we will be in trouble.” (Financial Times 2017).
This would be a retrograde step indeed and would take us back to the first instances of robots, as seen here in this image from a play by Czech writer Karel Čapek who introduced the word robot in 1920 to describe a race of artificial humans in a futurist dystopia.
To sum things up, we need to make these three things happen:
- Use AI to tackle educational challenges
- Prioritize the development of our uniquely human intelligence
- Educate people about AI
To do this we need partnerships between educational stakeholders to build capacity.
Partnerships and the Golden Triangle
There are 3 ways AI can enhance Learning and Teaching
- Tackle Educational Challenges using AI
- Prioritize Human Intelligence
- Educate people about AI: Attention to Ethical AI for Education is essential
Partnerships are the only way we can achieve this, partnerships of the type that we build through the EDUCATE programme.
These partnerships are to generate the golden triangle that is the foundation of impactful high-quality educational technology (including AI) design and application.
The idea of the Golden Triangle formed at a meeting in January 2012 when I was talking with my research colleagues Mike Sharples and Richard Noss, along with Clare Riley from Microsoft and Dominic Savage from BESA – we had met up with some educators and were puzzling about why the UK Educational Technology business was not better connected to researchers and educators.
This was the birth of the triangle, the points of which are:
- EdTech developers
- Researchers, and
- Educators
All of whom need to be brought together to develop and apply the best that technology can provide for education. The triangle is golden, because it is grounded in data.
It is a triangle, because it connects the three key communities, the people who:
- Use the technology,
- Build the technology, and
- Know how to evidence the efficacy of the technology for learning and or teaching.
This golden triangle is at the heart of what needs to be done for AI to be designed and used for education in ways that will support our educational needs.
It is the triangle at the heart of the partnerships that engage the AI developers, most of whom do not understand learning or teaching, with the educators, most of whom do not understand AI, and the researchers who understand AI and learning and teaching.
It is the co-design partnership that will drive better AI for use in education, more educated educators who can drive the changes to the way we teach and learn that are required for the fourth industrial revolution and also help their students understand AI, and more educationally savvy AI developers
The Reality and the Potential of AI is that AI is smart, but humans are and can be way smarter.
Rose Luckin, Professor of Learner Centred Design, UCL Knowledge Lab
First published on The Knowledge Illusion, this article reviews AI and Education: the Reality and the Potential
In the first article “Intelligent Tutoring Systems: Data is the New Oil” Rose covered the way AI can support teaching and learning.
The next article, “Educating people about Artificial intelligence, so that they can use it safely and effectively” dicussed helping people understand enough about AI to know the basics of what it can and cannot achieve.
Responses