Machines that Think
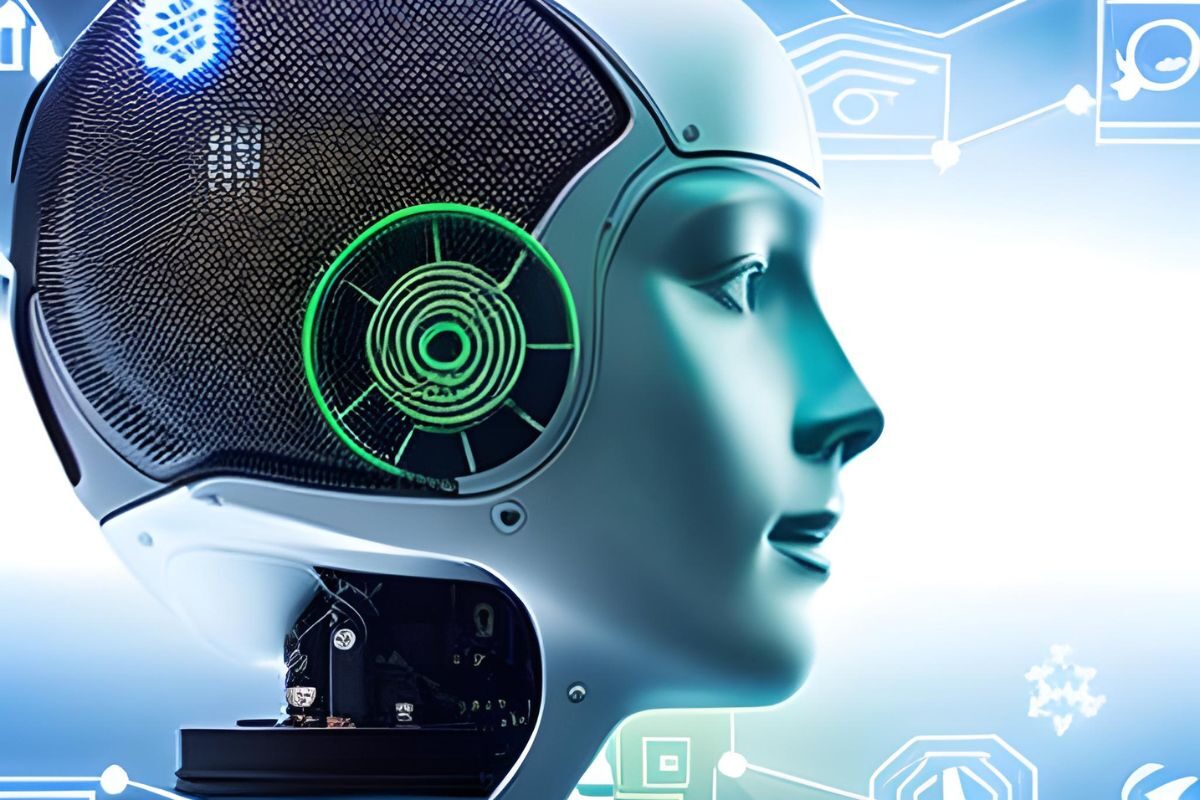
This 260-page paperback in an Instant Expert series (1) holds eight chapters whose titles may well capture the spirit of a machine-learning age, for instance, Chapter 3: Anything You Can Do, Chapter 4: Matters of Life and Death, Chapter 5: Into the Unknown, and Chapter 6: Machine that Create. The pace and promise of Artificial Intelligence has quickened since early exercises in designing and applying automatons; not only are the best practitioners of GO beaten, competitors can be driven by machines to their matches as passengers, hands free of the steering wheel.
Exemplified by IBM’s Watson for medical diagnosis, readers are informed of important research initiatives within several sectors, but this subject didn’t exactly launch on all four thrusters, rather it ‘got off to a shaky start’. A mathematical approach named Symbolic Reasoning in which ideas and concepts were represented by symbols such as words and sentences that can be handled by the laws of logic so far hasn’t fulfilled a hope of becoming intelligent.
Interesting – though possibly confusing – how the name ‘Symbolic Reasoning’ might refer to 1960s commercial programming which also turns the programmer’s ideas into words and sentences via a logical set of (old school) instructions: IF STOCK OF ZBOLTS IS LESS THAN 60 THEN PERFORM ZB-ORDER-ROUTINE.
Chapter 2 introduces success stories of Google, Palem, and Animat, – and also helps categorize the old and new branches of Artificial Intelligence. Bold pioneering aims of the1956 Dartmouth Conference – text understanding, control of robots, and machine learning – relied on the so-called top-down logical computer programs which proved unsuited to the ‘messiness of the real world’.
They had proved practical in areas such as corporate payroll because the problem to be run on computers was complex but fathomable in logical relations between months/hours worked, rates of pay, taxation, and pension deductions. If it had been unfathomable no employee could have ever been encouraged to join and ascend the payroll department’s career ladder!
And today it seems reasonable to describe a computer program for payroll or stock control written since the sixties as a traditional instance of Artificial Intelligence: either program is an abstract device that performs a complex task by means of binary strings. Crucially, more recent experts don’t know how the new systems do what they do, even though its language is apparently composed of ‘simple statistical tricks’ (p29). A new wave of AI is radical because of the mysterious way it applies statistics to immense volumes of data – a paradigm shift in the industry.
For example, if Wendy is a new customer the software will generalize and attempt to predict her future spending according to factors like age, location, and costs. We can’t see the ‘why’ within masses of data and ‘chaos of correlations’ but must be content that it does work: explanations don’t matter. Does affordability matter? Will advanced driverless vehicles, educational software and medical diagnosis routines be widely available to tens of thousands of UK institutions or constrained because the fledgling industry itself needs to develop through time, i.e., earn a good ROI from customers?
The origins of the applied statistics are not revealed in this book and may have been developed earlier for well-discussed fields like botany or politics (I guess both subjects are rich in data, the latter seldom smelling like roses and trickier to unravel). Phrases like ‘intelligence in the data’ and ‘you get it to learn to do things’ suggest the need for a carefully composed set of instructions to point statistical software towards data where they can extract findings.
This must be done because computers aren’t naturally curious. Maybe their minds are ‘not like ours’ because they don’t qualify as minds at all. The second chapter also discusses how symbolic AI (manually describing everything) is being linked to artificial neural networks at Imperial College London. A human brain holds 100 trillion synapses connecting 100 billion neurons, which can change state several times a second. A supercomputer has 100 trillion bytes of memory – and is faster.
Again, in essays, the terminology of memory, connections and networks moves effortlessly between the very different worlds of multifarious Homo sapiens and inanimate symbol-crunching modern technology. But Wendy’s memory is occasionally fickle, forgetful, and emotional: disinterested in football, she overlooks a request to record Match of the Day but remains heartbroken two days after a new boyfriend doesn’t turn up for their planned date at the town’s cinema. Computers can outsmart us in chess, Go, steering cars tidily into parking bays, and flying large planes via autopilot, but they cannot understand the agonies and ambition of being fallible Homo sapiens.
Gadgets described in the book perform a restricted range of tasks, but equally important are organizational personnel rethinking troublesome aspects of their milieu, whether, say, local difficulties in retaining students/staff or broader issues over congestion, accommodation, and delays in factory production lines?
Just as AI packages have complex (apparently unfathomable) internal logical workings, personnel troubled by an uncertain status quo might benefit from support via explicit logical devices that permit responses such as, ‘Yes, that’s what we need to discuss!’ Or ‘No, that sketch is too radical for management team – be more conservative!’ Steeped in high-speed symbol processing, AI seems some distance from helping to arrange this type of enquiry into the nature of the problem.
What if it isn’t obvious? One image selected from ten thousand recorded images of a railway station platform won’t necessarily indicate what will follow in the next five minutes: a train arriving, a train departing, passengers getting off, passenger getting on, emergency doctor racing towards the train, police with dogs getting on, cleaners getting off, the arrival of football hooligans, snow, sleet, fog, etc.
The book’s glossary (p263) defines Narrow Artificial Intelligence as AI that is good at a specific task only, such as picking out faces in a crowd or driving a car, while General Artificial Intelligence can carry out a wide range of tasks with human-like ability. But doesn’t the best driving belong in the second category, coping as it eventually must with fast-moving and occasionally careless motorway drivers, inclement weather, traffic signals, cyclists’ expectations, emergencies, and maybe errors made by pedestrians?
So, recalling the book’s title, Machines that Think, is something which machines by definition can’t do, and, how hype over AI currently matches that of the web’s early days, and the way the author’s closing paragraphs call for a reality check, our extinction by some style of super-intelligence being only one of several ‘esoteric possibilities’, how does AI help alleviate society’s esoteric yet close-to-home, problematic possibilities?
1) Douglas Heaven and six academic contributors; Machines that Think, New Scientist, John Murray Learning, 2022
By Neil Richardson
Responses